Read about local events and news from IDEAL. Sign up for our Newsletter to stay up to date with events and announcements about IDEAL.
New NSF awards will advance theoretical foundations of data science research through interdisciplinary collaborations
Advancing the Theoretical Foundations of Data Science- IDEAL received a five-year, $10 million Phase II award from NSF
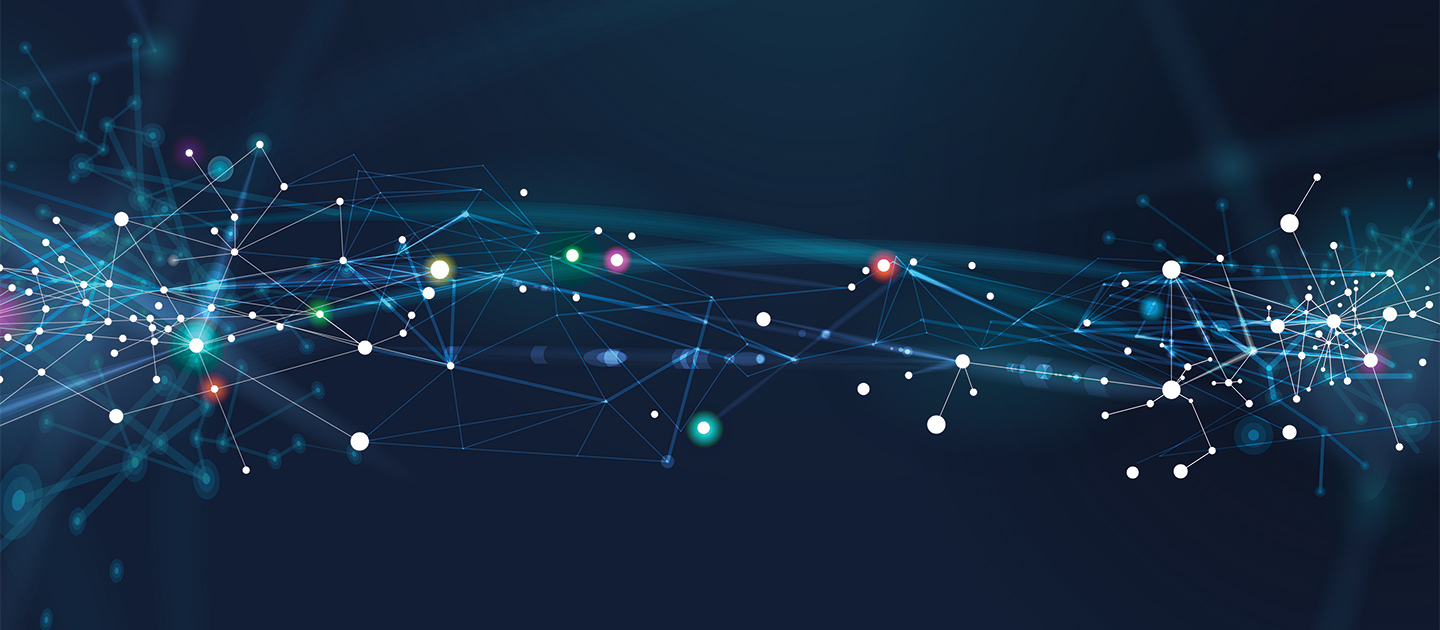
Accelerating transformative advances in the theoretical foundations of data science, the Institute for Data, Econometrics, Algorithms, and Learning (IDEAL) unites a team of faculty, students, postdoctoral fellows, and researchers to study machine learning and optimization, high-dimensional data analysis and inference, and emerging topics including reliability, interpretability, privacy, and fairness.
The National Science Foundation (NSF) announced that IDEAL is one of two institutes receiving Harnessing the Data Revolution (HDR): Transdisciplinary Research in Principles of Data Science (TRIPODS) Phase II awards. The five-year, $10 million Phase II project combines and builds upon the complementary strengths of two Phase I TRIPODS institutes — IDEAL Phase I (2019-2022) and the University of Illinois at Chicago (UIC) Foundations of Data Science Institute.
Collaborating across disciplines and institutions
More than 60 investigators in computer science, economics, electrical engineering, law, mathematics, operations research, and statistics will collaborate across Northwestern, Google Research, the Illinois Institute of Technology (IIT), the Toyota Technological Institute at Chicago (TTIC), UIC, and the University of Chicago.
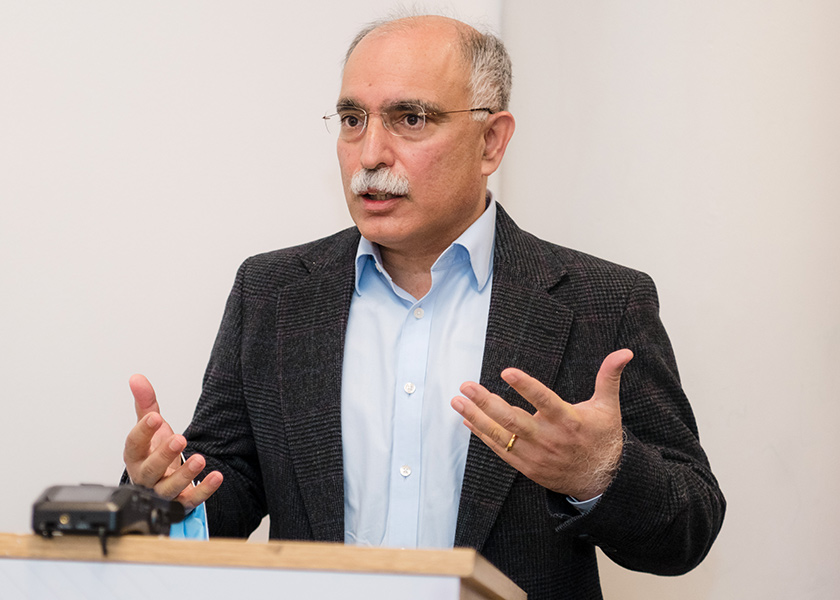
“Leveraging the power of data and computation to solve grand challenge problems of this century is central to this exciting new project,” said Samir Khuller, Peter and Adrienne Barris Chair of Computer Science and co-principal investigator of IDEAL. “In Phase I, we established a strong and collaborative working relationship with our partners at TTIC and the University of Chicago. The continued support from NSF to establish the larger institute with all of the Chicago-area research universities shows confidence in our progress over the last three years and we hope to be a lot more than the sum of our parts.”
IDEAL site director Aravindan Vijayaraghavan, associate professor of computer science and (by courtesy) industrial engineering and management sciences at Northwestern Engineering, will lead the Northwestern arm of the Phase II institute.
“We are very grateful to the NSF for making such a large investment in basic research related to data science foundations through the IDEAL institute,” Vijayaraghavan said. “This is a truly interdisciplinary undertaking. Our institute brings together exceptional researchers both in core data science disciplines and in fields outside the traditional scope.”
The Northwestern team also includes:
- Eric Auerbach, assistant professor of economics at the Weinberg College of Arts and Sciences
- Antonio (Tuca) Auffinger, professor of mathematics at Weinberg
- Randall Berry, John A. Dever Chair of Electrical and Computer Engineering
- Federico Bugni, professor of economics at Weinberg
- Ivan Canay, HSBC Research Professor of Economics at Weinberg
- Julia Gaudio, Patrick and Amy McCarter Fellow and research assistant professor of industrial engineering and management sciences and (by courtesy) computer science
- Ben Golub, associate professor of economics at Weinberg and computer science
- Dongning Guo, professor of electrical and computer engineering and (by courtesy) computer science
- Jason Hartline, professor of computer science
- Joel Horowitz, Charles E. and Emma H. Morrison Professor of Economics at Weinberg
- Jessica Hullman, Ginni Rometty Professor of Computer Science
- Annie Liang, assistant professor of economics at Weinberg and the Karr Family Assistant Professor of Computer Science
- Konstantin Makarychev, professor of computer science
- Jorge Nocedal, Walter P. Murphy Professor of Industrial Engineering and Management Sciences and (by courtesy) professor of engineering sciences and applied mathematics and director of the Center for Optimization and Statistical Learning
- Daniel W. Linna Jr., senior lecturer and director of law and technology initiatives
- Zhaoran Wang, assistant professor of industrial engineering and management sciences and (by courtesy) computer science
- Ermin Wei, assistant professor of electrical and computer engineering and of industrial engineering and management science
“It is exciting to participate in this project, which brings together Chicago-area researchers in diverse areas, including computer science, economics, electrical engineering, and industrial engineering,” Berry said. “This multidisciplinary expertise is essential to further the foundation of data science.”
IDEAL leadership at partner organizations includes Avrim Blum, professor and chief academic officer at TTIC; Jinqiao (Jeffrey) Duan, professor of applied mathematics at IIT; Chao Gao, assistant professor in statistics at the University of Chicago; and Lev Reyzin, professor of mathematics, statistics, and computer science at UIC.
Expanding participation in data science
Through research, training, and outreach activities, IDEAL aims to broaden local and national participation in data science, build lasting research and educational infrastructure, and bridge the theoretical foundations of data science and the practice of data science in industry and applied science.
“We aim to establish the Chicago-area as a center of excellence in the foundations of data science,” Khuller said. “Along with our unique partnerships with Discovery Partners Institute, P33, and industrial partners such as Google, we hope to engage students at all levels in our research and training efforts. I am truly excited to be part of this collaboration and we look forward to many years of impactful research.”
IDEAL’s five-year plan will lead to new theoretical frameworks, models, mathematical tools, and algorithms for analyzing high-dimensional data, inference, and learning.
“Data science is crucial for decision-making in almost every domain of business and engineering,” said Dean Julio M. Ottino. “The IDEAL partner organizations will both further the study of foundational problems of data science and engage a broader audience with this critical field.”
Building on Phase 1 foundations
The institute’s research focus centers around interdisciplinary topics, including deep learning and optimization, reinforcement learning, data science with strategic agents, trustworthy and reliable data science, and interpretability, privacy, and fairness, which will be the theme of special programs held each fall and winter.
As co-directors of IDEAL Phase 1, Vijayaraghavan and Hartline introduced thematically focused “special quarters,” to foster interdisciplinary, inter-institute collaborative research, such as the spring 2021 Special Quarter on Data Science and Law, co-organized with Linna.
“The program was incredibly productive,” Hartline said. “We ran a virtual graduate seminar, two ongoing research projects were accepted to the Second ACM Symposium on Computer Science and Law, and an international special interest group continues to hold virtual monthly meetings around a technical program organized by rotating hosts.”
Engaging students, postdocs, and researchers at all career stages, IDEAL Phase 2 will coordinate weekly research meetings, brainstorming sessions, graduate courses, co-mentoring, workshops, and reading groups. IDEAL’s first special program, “Machine Learning and Logic,” will launch in winter 2023.
“We are excited about the research activity that the institute will generate both at Northwestern and in the broader Chicago area, through new research projects and interdisciplinary collaborations, coordinated graduate coursework, short-term research visitors and workshops,” Vijayaraghavan said.
Broadening educational pathways
To broaden and strengthen educational pathways, IDEAL will also lead activities targeted to undergraduate students, high school students, and the broader public. IDEAL will connect undergraduate students from underrepresented backgrounds and from institutions lacking resources for research to training opportunities within member institutions. The institute will also launch a program to prepare undergraduates to apply for and thrive in NSF-funded Research Experiences for Undergraduates (REU) sites.
In addition, IDEAL will coordinate undergraduate research workshops, data science workshops for high school science and math teachers, and public events, such as lectures and programming at the Museum of Science and Industry.
“The new 2022 TRIPODS awards address foundational challenges in data science at the core of data-driven discovery and decision making,” said NSF Division Director for Computing and Communication Foundations (CCF) Dilma Da Silva. “CCF is pleased to be able to support these impactful projects.”
article link: https://www.mccormick.northwestern.edu/news/articles/2022/07/advancing-the-theoretical-foundations-of-data-science/
Annie Liang Receives NSF CAREER Award
The award will support research at the intersection of economic theory and computer science
Northwestern’s Annie Liang has received a Faculty Early Career Development Program (CAREER) award from the National Science Foundation (NSF), its most prestigious honor for junior faculty members.Liang is an assistant professor of economics at Northwestern’s Weinberg College of Arts and Sciences and the Karr Family Assistant Professor of Computer Science at Northwestern Engineering.
The CAREER program supports the early-career development of academic role models dedicated to outstanding research, inspired teaching, enthusiastic learning, and disseminating new knowledge to a broad audience. The award is intended to build a firm foundation for a lifetime of contributions to research, education, and their integration.
Liang will receive $408,451.00 over five years from NSF’s Division of Social and Economic Sciences for her project titled “Information, Algorithms, and Learning.” The grant will fund projects at the intersection of economic theory and computer science, which explore the opportunities and the dangers presented by algorithmic approaches within economic settings. Liang will develop new economic models of algorithmic decision-making, and new methodologies for the interpretation of economic data.
“Machine learning algorithms are increasingly used to guide economic predictions and decisions.” Liang said. “We need to better understand how to deploy these algorithms in a thoughtful way, and what the broader social ramifications of these algorithms are.”
The project has three components, which Liang will pursue with different groups of coauthors. In the first project, Liang plans to characterize the welfare implications of algorithmic predictions, focusing on the tradeoffs between goals such as the accuracy versus the “fairness” of the algorithm. In the second, Liang will propose new methods for understanding how predictions made by ML algorithms that are opaque and uninformative about underlying economic forces — so-called black box methods — differ from those made by economic models. In particular, Liang will compare how well these methods generalize to new domains. Finally, Liang will model information flow on social media platforms when the social norms that govern acceptable expression are in flux, characterizing when and how expressed views systematically differ from the true distribution of opinions.
By developing new models, proving new theoretical results, and proposing new statistical measures, these project will advance the research frontier in economic theory. The work is fundamentally interdisciplinary in nature, building on ideas from the neighboring areas of computer science and statistics.
The CAREER project also includes an education component focused on developing curriculum for new courses at the intersection of economics and computer science.
“It’s extremely encouraging to learn that the NSF panel and reviewers are excited, as I am, about understanding the economic and social implications of machine learning,” Liang said. “I look forward to continuing to explore problems in this area.”
Prior to joining Northwestern in 2021, Liang was an assistant professor of economics at the University of Pennsylvania, and a postdoctoral researcher at Microsoft Research-New England. She earned a PhD in economics from Harvard University in 2016.
Showcasing the Work and Perspectives of Early-Career Researchers
Postdoctoral scholars in computer science organized a virtual workshop featuring presentations by junior researchers in theoretical computer science
On December 9-10, the Northwestern CS Theory Group and the Institute for Data, Econometrics, Algorithms, and Learning (IDEAL) co-hosted the 2021 Junior Theorists Workshop.
Sami Davies and Sheng Yang, postdoctoral scholars in computer science at Northwestern Engineering, organized the virtual workshop focused on the work and perspectives of junior researchers in theoretical computer science.Davies is a Computing Innovation Fellow and studies beyond worst-case analysis with IDEAL. She earned a PhD in mathematics from the University of Washington, an MS in mathematics from the University of Illinois at Chicago, and a BS in mathematical sciences from Carnegie Mellon University.
Yang investigates graph algorithms, discrete optimization, and stochastic optimization and is focused on classical and emerging, cloud-based scheduling problems. He earned a PhD in computer science from the University of Maryland, College Park, and BE in computer science from Tsinghua University.”This workshop allows our graduate students and postdocs to learn from some of the most talented junior researchers across the globe and enables new collaborations in theoretical computer science,” said Samir Khuller, Peter and Adrienne Barris Chair of Computer Science. “The speakers were chosen via an extremely competitive process from a long and distinguished list of nominees.”
On the first day of the event, guest speakers presented on topics including dynamic data structures, sublinear-time algorithms and arboricity, multidimensional packing problems, approximation algorithm for metric traveling salesman problem, smoothed analysis of approximate diagonalization, and approximating constraint satisfaction problems.
Day 1 presenters:
- Jess Banks, PhD candidate in mathematics at the Simons Institute for the Theory of Computing and University of California, Berkeley
- Chi-Ning Chou, PhD student in the theory of computation group at Harvard University
- Talya Eden, postdoctoral fellow at the Foundations of Data Science Institute, jointly affiliated with Boston University and MIT
- Maximilian Probst Gutenberg, postdoctoral fellow in computer science at ETH Zürich
- Nathan Klein, PhD student in computer science and engineering at the University of Washington
- Sai Sandeep Pallerla, PhD student in algorithms and complexity theory at Carnegie Mellon University
In addition, Yingkai Li, a PhD student in computer science at Northwestern Engineering, and Shravas Rao, postdoctoral fellow in computer science at Northwestern Engineering, presented Northwestern spotlight talks.
Li is interested in information design, game theory, and online algorithms. He discussed an endogenous information mechanism design model in which the designer’s choices about the algorithm directs how much and what type of information the agents choose to acquire.
Rao studies theoretical computer science and presented his work related to restrictions on the slice theorem. He is interested in researching the relationship between the slice and the concept of high dimensional expanders.
“Outstanding junior researchers from all areas of theoretical computer science spoke about their exciting research and presented novel algorithms, techniques, and data structures to the workshop attendees,” said Konstantin Makarychev, professor of computer science in Northwestern Engineering.
The second day of the workshop featured discussions on algorithms for uniformly sampling convex bodies, approximate solvers for linear programs based on interior-point methods, barriers to proving depth-separation results in neural networks, bilateral trade, difference estimators for data stream computation, and universal estimators for symmetric property estimation.
Day 2 presenters:
- Aditi Laddha, PhD student in algorithms, combinatorics, and optimization at Georgia Institute of Technology
- Bento Natura, PhD student in mathematics at the London School of Economics
- Kiran Shiragur, PhD student in management science and engineering, Stanford University
- Gal Vardi, postdoctoral fellow in computer science and applied mathematics at the Weizmann Institute of Science
- Kangning Wang, PhD student in computer science at Duke University
- Samson Zhou, postdoctoral researcher in computer science at Carnegie Mellon University
“Postdocs Sami Davies and Sheng Yang, did a masterful job in organizing the event, introducing speakers and assigning session chairs, and making a wonderful and relaxed schedule,” Khuller said.
Jason Hartline, professor of computer science, and Aravindan Vijayaraghavan, associate professor of computer science in Northwestern Engineering, are co-directors of IDEAL and co-organize the CS Theory Group Quarterly Theory Workshop series. IDEAL is an interdisciplinary, collaborative team of researchers from Northwestern, Toyota Technological Institute at Chicago, and the University of Chicago studying the foundations of data science.
Additional CS Theory Group members include:
- Benjamin Golub, associate professor of computer science and of economics at Northwestern’s Weinberg College of Arts and Sciences
- Samir Khuller, Peter and Adrienne Barris Chair of Computer Science
- Annie Liang, Karr Family Assistant Professor of Computer Science and of economics at Weinberg
- Konstantin Makarychev, professor of computer science
- Xiao Wang, assistant professor of computer science
IDEAL is a Harnessing the Data Revolution (HDR) Transdisciplinary Research in Principles of Data Science (TRIPODS) institute supported by the National Science Foundation under award CCF 1934931.
article link: https://www.mccormick.northwestern.edu/computer-science/news-events/news/articles/2022/showcasing-the-work-and-perspectives-of-early-career-researchers.html
Hartline Named 2021 Economic Theory Fellow
SAET recognizes substantial contributions to the field of economic theory
Northwestern Engineering’s Jason Hartline has been named a Society for the Advancement of Economic Theory (SAET) 2021 Economic Theory Fellow.
“It is really fantastic that work by computer scientists in the interface between computer science and economics is being recognized within mainstream economic theory,” said Hartline. “The other computer scientists who have been similarly recognized — Michael Kearns at the University of Pennsylvania, Nimrod Megiddo at IBM Almaden, Noam Nisan at Hebrew University, Christos Papadimitriou and Tim Roughgarden at Columbia University, and Moshe Tennenholtz at Technion — are all distinguished pioneers of the field.”
Hartline applies design and analysis methodologies from computer science to explain and improve the behavior and outcomes of complex economic systems.
In his graduate textbook Mechanism Design and Approximation, Hartline introduces the theory of approximation to auction theory and mechanism design. The approach quantifies the tradeoff between optimal outcomes and other desirable properties such as simplicity, robustness, computational tractability, and practicality.
“Professor Hartline is a leader in his field of mechanism design. This award, coming on the heels of his recent SIGEcom test of time award, is a testament to the amazing respect he commands in computer science and economics and how the two fields have co-evolved,” said Samir Khuller, Peter and Adrienne Barris Chair of Computer Science in the McCormick School of Engineering. “Northwestern CS is the best place to do interdisciplinary research like this. I am really proud to call him my colleague and friend.”
The SAET Fellowship Committee considers nominees’ publication record, editorial board service, graduate student training, and honorary, scientific, educational, and professional accomplishments.
“I think models and methods of analysis from computer science have a tremendous potential for impact in economics and that we have only just started to see this potential be realized,” Hartline said.
Hartline is a co-director of the Institute for Data, Econometrics, Algorithms, and Learning (IDEAL), a team of interdisciplinary investigators at Northwestern, Toyota Technological Institute at Chicago, and the University of Chicago that studies the theoretical foundations related to high dimensional data analysis, data science in strategic environments, and machine learning and optimization.
He also co-organizes the Northwestern CS Theory Group Quarterly Theory Workshop series and the Northwestern CS+Economics Quarterly Workshop.
Hartline is an associate editor of the Journal of Economic Theory and the president and cofounder of Virtual Chair Inc., a virtual conference organizing platform.
Founded in 1991, SAET’s mission is to advance knowledge in theoretical economics and to facilitate communication among researchers in economics, mathematics, game theory, and other fields. The organization publishes the journals Economic Theory and the Economic Theory Bulletin.
article link: https://www.mccormick.northwestern.edu/computer-science/news-events/news/articles/2022/hartline-named-2021-economic-theory-fellow.html
Alumni Profile: Yiding Feng, Microsoft Research Lab – New England
Yiding Feng (PhD ’21) is a postdoctoral researcher at Microsoft Research Lab – New England (MSR). He earned his PhD in computer science with a focus on CS + Economics research in mechanism design, online algorithms, and information design under the supervision of Jason D. Hartline, professor of computer science at Northwestern Engineering.While he was an undergraduate student in computer science in the Association of Computing Machinery Honors Class at Shanghai Jiao Tong University, China, Feng was a research intern at Microsoft Research Asia and Hong Kong University.
We asked him about his research interests, impactful collaborative experiences at Northwestern CS, and his advice for current students.
Why did you decide to pursue your PhD at Northwestern CS?
I wanted to work with Professor Jason Hartline in the interdisciplinary CS + Economics program. I also really enjoyed the culture and atmosphere of Northwestern CS. The whole department is expanding quickly. I am impressed by the great passion faculty and staff have for research.
Could you describe your research interests in one sentence?
I study how to design and evaluate economic systems and policies from a computer science perspective.
What was the focus of your thesis project?
I approached auction design — a classic problem in microeconomics related to the allocation of resources to strategic agents who have private preferences over those resources — as a multi-party computation problem. Auction design is foundational to a vast number of practical applications, including spectrum auctions, electricity markets, wireless sensor networks, e-commerce websites, and online advertising.
Economics literature provides a good answer to one fundamental question in multi-party computation — what are the incentives to do it correctly? However, the economics and computer science disciplines approach the second major question — who should be conducting which part of the computation? — with different high-level guidelines.
The end-to-end argument, a long-standing principle in CS system design, suggests the computation should be done in a decentralized manner. On the other hand, the field of mechanism design in economics favors systems in which the entire computation is centralized, and participants report a portion of optimized input data. In my thesis, I address this discrepancy by arguing the decentralization principles from CS system design are beneficial even in purely economic terms.
In online advertising platforms, for instance, advertisers bid for ad slots using different auction formats implemented to determine allocation and payments. In truthful mechanism formats, advertisers prefer to set their bids equal to the true value of the ad slots and the computation is centralized. In non-truthful mechanism formats, the computation is decentralized, and advertisers compute the level they underbid to the true value while the auction center determines allocation.
Non-truthful mechanisms are prevalent. Google Ads Manager, for example, recently shifted their auction format from second-price auction (a truthful mechanism) to first-price auction (a non-truthful mechanism). I developed new analysis tools to analyze and design non-truthful mechanisms, and provided theoretical justification on why non-truthful mechanisms are better from a robustness standpoint.
What are some examples of collaborative experiences at Northwestern that were impactful to your research?
In 2019, during my third year, I visited Stanford University for eight months at the recommendation of Professor Hartline. My collaboration with Stanford faculty members Amin Saberi, professor of management science and engineering, and Rad Niazadeh, postdoctoral fellow in computer science, was extremely impactful because it helped me expand my research interests to projects at the intersection of computer science and operations research.
In spring 2020, I participated in the special quarter on inference and data science on networks hosted by the Institute for Data, Econometrics, Algorithms, and Learning and started a remote collaboration with John Birge, Hobart W. Williams Distinguished Service Professor of Operations Management, and Ozan Candogan, associate professor of operations management, both at the University of Chicago Booth School of Business. We worked on a project which studied how to use mobility data to design the targeted lockdown policy for COVID-19. The experience taught me how to make remote collaboration efficient and how to work on empirical studies.
What skills or knowledge did you learn in the PhD program that you think will stay with you for a lifetime?
One of the most important skills I learned is how to communicate and collaborate with people from different communities with diverse languages and approaches to research.
In the early stage of my PhD program, I took several courses from the Department of Economics and the Kellogg School of Management and, later, I started collaborating with researchers in those areas. The experience taught me how to have an open mind to embrace and work with people with different backgrounds.
What types of research and projects are you currently working on at Microsoft Research Lab – New England?
Research at MSR is similar to research in academia, but with more collaboration opportunities with product groups in industry. As a postdoc, I have the freedom to choose projects. Currently, I am working on projects related to auction theory, online algorithms, and information design that have potential applications to products and services at Microsoft such as Bing sponsor search and resource allocation in Azure.
I appreciate the skills I learned in the PhD program that enable me to have diverse collaborations with researchers in MSR, product teams in other departments, and other academic institutes.
What are your longer-term career goals?
My postdoc position ends in spring 2023. I will be on the job market in fall 2022 looking for jobs in both academia and industry. Due to my research background, I will apply for both faculty jobs in computer science and operations research.
What advice do you have for current Northwestern CS PhD students?
Enjoy your PhD program! You will have tough days and challenges to overcome. However, in the end, it is a great journey. Go Wildcats!
article link: https://www.mccormick.northwestern.edu/computer-science/news-events/news/articles/2022/alumni-profile-yiding-feng-microsoft-research-lab-new-england.html
Exploring New Directions on Robustness in Machine Learning
On November 16, IDEAL hosted a workshop focused on new directions on robustness in machine learning as part of the fall 2021 special quarter.
Machine learning systems are widely deployed to facilitate decision-making. They’re used for many tasks — ranging from image and speech recognition, to medical diagnostics and electronic health record data mining, to securities trading and financial fraud detection — making it vital for the systems to be reliable and secure against adversarial corruptions.
“It is well-known that machine learning algorithms and systems can be quite vulnerable to various kinds of errors, contamination and potentially adversarial corruptions,” said Aravindan Vijayaraghavan, associate professor of computer science in Northwestern Engineering.Robustness describes how much a system or algorithm can withstand and tolerate data errors, contamination, and misspecification during training, modeling, and testing phases.
Vijayaraghavan and Jason Hartline, professor of computer science in the McCormick School of Engineering, are co-directors of the Institute for Data, Econometrics, Algorithms, and Learning (IDEAL). Launched in 2019 by a team of interdisciplinary investigators at Northwestern, Toyota Technological Institute at Chicago, and the University of Chicago, IDEAL studies the theoretical foundations related to high dimensional data analysis, data science in strategic environments, and machine learning and optimization.
On November 16, IDEAL hosted a workshop focused on new directions on robustness in machine learning as part of the fall 2021 special quarter organized by Vijayaraghavan; Chao Gao, assistant professor of statistics at the University of Chicago; and Yu Cheng, assistant professor of mathematics at the University of Illinois at Chicago.
“During the fall special quarter, we studied some of the foundational questions about when and how we can design methods for machine learning and high-dimensional estimation that are robust and reliable,” said Vijayaraghavan.
Workshop speakers explored novel notions of robustness and the different challenges that arise in designing reliable and secure machine learning algorithms. Discussion topics included test-time robustness, adversarial perturbations, and distribution shifts.
Kamalika Chaudhuri, associate professor of computer science and engineering at the University of California San Diego, discussed the robustness of training algorithms to small, imperceptible perturbations to legitimate test inputs, or adversarial examples, that cause machine learning classifiers to misclassify.
Pranjal Awasthi, research scientist at Google, studies theoretical machine learning with a particular focus on designing robust algorithms for unsupervised learning.
Sébastien Bubeck, senior principal research manager for machine learning foundations at Microsoft Research, discussed joint research he conducted with Mark Sellke, a fourth-year graduate student in mathematics at Stanford University, that illustrates why robustness necessitates large neural networks
Aleksander Mądry, Cadence Design Systems Professor of Computing at MIT, presented a direct training data-to-output model that is a versatile framework for analyzing machine learning predictions.
Gautam Kamath, assistant professor of computer science at the University of Waterloo, specializes in robust statistics and data privacy. He surveyed different problems and results on differential privacy arising in the context of various statistical estimation settings.
Jinshuo Dong, IDEAL postdoctoral fellow, also helped organize the November event.
IDEAL’s next special quarter, “High Dimensional Data Analysis,” starts in spring 2022 and will include graduate courses, workshops, and reading groups. The spring quarter is being organized by Konstantin Makarychev, professor of computer science at Northwestern Engineering, and Yury Makarychev, professor of computer science at the Toyota Technological Institute at Chicago.
IDEAL is led by co-principal investigators from the three participating institutions. The Northwestern team also includes:
- Randall Berry, John A. Dever Chair of Electrical and Computer Engineering
- Dongning Guo, professor of electrical and computer engineering and (by courtesy) computer science
- Samir Khuller, Peter and Adrienne Barris Chair of Computer Science
- Zhaoran Wang, assistant professor of industrial engineering and management sciences and (by courtesy) computer science
- Eric Auerbach, assistant professor of economics at the Kellogg School of Management
- Ivan Canay, HSBC Research Professor of Economics at Kellogg
- Joel Horowitz, Charles E. and Emma H. Morrison Professor of Economics at Kellogg
IDEAL is a Harnessing the Data Revolution (HDR) Transdisciplinary Research in Principles of Data Science (TRIPODS) institute supported by the National Science Foundation under award CCF 1934931.
article link: https://www.mccormick.northwestern.edu/computer-science/news-events/news/articles/2022/exploring-new-directions-on-robustness-in-machine-learning.html
IDEAL in the News
Read recent media coverage of IDEAL.
June 6, 2020 | The New York Times
Coronavirus Shutdowns: Economists Look for Better Answers
November 15, 2019 | McCormick School of Engineering News
New Collaborative Institute Aims to Explore Theoretical Foundations of Data Science