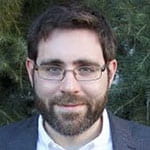
Eric Auerbach
(Northwestern, Economics)

Randall Berry
(Northwestern, ECE)
Prof. Berry’s research covers resource allocation problems that arise in networked systems ranging from communication networks to social networks. This work uses mathematical models to gain insights into such systems and draws on tools from stochastic modeling, optimization, economics and algorithms. Specific topics of current interest include developing distributed resource allocation technicques for wireless networks, dynamic spectrum sharing and wireless spectrum policy, understanding the role of incentives in network security and modeling learning and adoption in social networks.
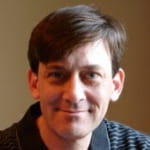
Avrim Blum
(TTIC, CS)

Ivan Canay
(Northwestern, Economics)
Ivan Canay’s research is in econometric theory, with specific interests in developing tests for assessing models that are partially identified. His most recent work includes methods for inference on individual components of parameters defined by moment inequalities, inference in models with few clusters via randomization tests, and inference under covariate adaptive randomization. He is currently an associate editor of the Journal of Econometrics, the Journal of Business and Economic Statistics, and the Econometrics Journal.

Ozan Candogan
(University of Chicago, Operations Management, Booth)
Ozan Candogan is an Associate Professor of Operations Management at Chicago Booth. Prior to joining Booth, he was an Assistant Professor at the Fuqua School of Business where he was a member of the Decision Sciences area. He received his Ph.D. and M.S. degrees in Electrical Engineering and Computer Science from the Massachusetts Institute of Technology.
Professor Candogan’s research is primarily motivated by the role that social and economic networks play in different operational settings. It has three complementary branches:
(i) Impact of networks on operational decisions.
(ii) Complex social and economic systems and new methodologies.
(iii) Pricing and revenue management problems in service systems.
Ozan Candogan was a finalist in the 2013 George Nicholson Student Paper Competition. He is also a recipient of the 2012 Microsoft Research Ph.D. Fellowship, and 2009 Siebel Scholarship. He teaches Operations Management, as well as a PhD course on Networks at Booth.

Jinshuo Dong
(Northwestern)
Jinshuo Dong joined IDEAL in 2020, after obtaining his PhD in applied math from the University of Pennsylvania under the supervision of Aaron Roth. Prior to that, he obtained a bachelor’s degree in mathematics from Peking University in 2014. The main theme of his research is various central limit phenomena in differential privacy. In addition to that, he is broadly interested in the mathematics of computation, information and intelligence.

Chao Gao
(University of Chicago, Statistics)
Chao Gao’s research interests are spread across nonparametric and high-dimensional statistics, network analysis, Bayes theory and robust statistics.

Dongning Guo
(Northwestern, ECE)
Dongning Guo’s research interests lie in communications, networking, information theory, and signal processing. His current projects concern next generation cellular networks, the Internet of Things, information theory of many-user systems, millimeter wave and full-duplex communication, as well as experimentation using software-defined radios. Dongning received his Master’s and Ph.D. in Electrical Engineering from Princeton University.

Varun Gupta
(University of Chicago, Operations Management, Booth)

Jason Hartline
(Northwestern, CS)
Prof. Hartline’s research introduces design and analysis methodologies from computer science to understand and improve outcomes of economic systems. Optimal behavior and outcomes in complex environments are complex and, therefore, should not be expected; instead, the theory of approximation can show that simple and natural behaviors are approximately optimal in complex environments. This approach is applied to auction theory and mechanism design in his graduate textbook Mechanism Design and Approximation (http://www.jasonhartline.com/MDnA/) which is under preparation.

Joel Horowitz
(Northwestern, Economics)
Joel Horowitz’s research is concerned with estimation and inference under weak assumptions about the process that generates the available data. It is also concerned with improving the accuracy of inference with samples of practical size and with using shape restrictions provided by economic theory to improve estimation and inference. He is a former co-editor of Econometrica and Econometric Theory, a Fellow of the Econometric Society and of the American Statistical Association, and an elected member of the International Statistical Institute.

Samir Khuller
(Northwestern, CS)
Samir Khuller is chair and Peter and Adrienne Barris Professor in the Department of Computer-Science at Northwestern University. Prior to joining Northwestern’s newly-established department of computer science in March 2019, Khuller spent twenty-nine years at the University of Maryland College Park where he held the Elizabeth Stevinson Iribe Chair, and spearheaded the development of the Brendan Iribe Center for Computer Science and Engineering.
Khuller’s research interests are centered on graph algorithms, discrete optimization and computational geometry. He has published over 200 journal and conference papers as well as book chapters on these topics. He is a recipient of the National Science Foundation’s Career Development Award, a Google Research Award as well as the University of Maryland’s Distinguished Scholar Teacher Award. In 2016, his work on Connected Dominating Sets (with Sudipto Guha) received the European Symposium on Algorithms inaugural Test of Time Award.
Khuller has served as editor for several journals including Algorithmica, the International Journal on Foundations of Computer Science and Networks. He has also served on numerous program committees including SODA 1997, APPROX 1999, APPROX 2000 (chair), STOC 2003, PODS 2006, SODA 2007, APPROX 2010, ESA 2010, STOC 2013, SPAA 2017. A member of the ESA Steering Committee (2012-2016), he chaired the 2019 MAPSP Scheduling Workshop and will serve as the Chair of SIGACT from 2018-2021.
Khuller received his M.S and Ph.D from Cornell University in 1989 and 1990, respectively, under the supervision of Vijay Vazirani. His completed his undergraduate degree in computer science at the Indian Institute of Technology (Kanpur) in 1986.

Konstantin Makarychev
(Northwestern, CS)

Yury Makarychev
(TTI Chicago, CS)
Yury Makarychev received his MS degree in Mathematics from Moscow State University in 2001 and his PhD degree in Computer Science from Princeton University in 2008. He spent the following two years as a Postdoctoral Researcher at Microsoft Research in Redmond, WA and Cambridge, MA.
Yury’s research interests include combinatorial optimization, approximation algorithms, semi-definite programming, and low-distortion metric embedding. He has recently worked on developing approximation algorithms for unique games, constraint satisfaction, graph partitioning, and vertex ordering problems, investigating tradeoffs between local and global properties of metric spaces, and studying lift-and-project hierarchies of mathematical relaxations.
Dr. Makarychev also has a personally maintained website which can be found at http://www.ttic.edu/makarychev.

Nathan Srebro
(TTI Chicago, CS)
Dr. Srebro is interested in statistical and computational aspects of machine learning, and the interaction between them. He has done theoretical work in statistical learning theory and in algorithms, devised novel learning models and optimization techniques, and has worked on applications in computational biology, text analysis and collaborative filtering. Before coming to TTIC, Dr. Srebro was a postdoctoral fellow at the University of Toronto and a visiting scientist at IBM Research.
Dr. Srebro also has a personally maintained website which can be found at http://www.ttic.edu/srebro.

Ali Vakilian
(TTI Chicago)
Ali Vakilian received his Ph.D. degree from EECS department at MIT in 2019. Prior to MIT, he graduated from UIUC with M.S. degree in Computer Science. Before joining IDEAL, He spent a year as a postdoc at University of Wisconsin-Madison.
He has a broad research interest in streaming/sketching algorithms, learning-based (aka data-driven) algorithms and algorithmic fairness.
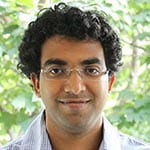
Aravindan Vijayaraghavan
(Northwestern, CS)
Aravindan Vijayaraghavan’s research interests are broadly in the field of Theoretical Computer Science, particularly, in designing efficient algorithms for problems in Combinatorial Optimization and Machine Learning. He is also interested in using paradigms that go Beyond Worst-Case Analysis to obtain good algorithmic guarantees.

Zhaoran Wang
(Northwestern, IEMS)
I am an assistant professor in the Departments of Industrial Engineering & Management Sciences and Computer Science (by courtesy) at Northwestern University (since 2018). I am affiliated with the Centers for Deep Learning and Optimization & Statistical Learning.
The long-term goal of my research is to develop a new generation of data-driven decision-making methods, theory, and systems, which tailor artificial intelligence towards addressing pressing societal challenges. To this end, my research aims at:
- making deep reinforcement learning more efficient, both computationally and statistically, in a principled manner to enable its applications in critical domains;
- scaling deep reinforcement learning to design and optimize societal-scale multi-agent systems, especially those involving cooperation and/or competition among humans and/or robots.
With this aim in mind, my research interests span across machine learning, optimization, statistics, game theory, and information theory.